David B. Dunson, Arts and Sciences Distinguished Professor of Statistical Science
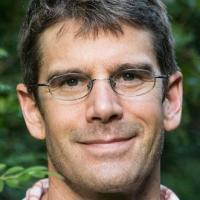 My research focuses on developing new tools for probabilistic learning from complex data - methods development is directly motivated by challenging applications in ecology/biodiversity, neuroscience, environmental health, criminal justice/fairness, and more. We seek to develop new modeling frameworks, algorithms and corresponding code that can be used routinely by scientists and decision makers. We are also interested in new inference framework and in studying theoretical properties of methods we develop.
Some highlight application areas: (1) Modeling of biological communities and biodiversity - we are considering global data on fungi, insects, birds and animals including DNA sequences, images, audio, etc. Data contain large numbers of species unknown to science and we would like to learn about these new species, community network structure, and the impact of environmental change and climate.
(2) Brain connectomics - based on high resolution imaging data of the human brain, we are seeking to developing new statistical and machine learning models for relating brain networks to human traits and diseases.
(3) Environmental health & mixtures - we are building tools for relating chemical and other exposures (air pollution etc) to human health outcomes, accounting for spatial dependence in both exposures and disease. This includes an emphasis on infectious disease modeling, such as COVID-19.
Some statistical areas that play a prominent role in our methods development include models for low-dimensional structure in data (latent factors, clustering, geometric and manifold learning), flexible/nonparametric models (neural networks, Gaussian/spatial processes, other stochastic processes), Bayesian inference frameworks, efficient sampling and analytic approximation algorithms, and models for "object data" (trees, networks, images, spatial processes, etc).
- Contact Info:
Teaching (Fall 2024):
- STA 941.01, BAYESIAN NONPARAMETRICS
Synopsis
- Old Chem 201, MW 10:05 AM-11:20 AM
- Office Hours:
- Thurs 9-10am
- Education:
Ph.D. | Emory University | 1997 |
PhD | Emory University | 1997 |
B.S. | Pennsylvania State University | 1994 |
- Specialties:
-
Bayesian Statistics
Complex Hierarchical and Latent Variable Modelling Nonparametric Statistical Modelling Model Selection Statistical Modeling
- Research Interests: Nonparametric Bayes, Latent variable methods, Model uncertainty, Applications in epidemiology & genetics, Machine learning
Current projects:
Nonparametric Bayes methods for conditional distributions, Semiparametric methods for high-dimensional predictors, New priors for functional data analysis, Borrowing information across disparate data sources, Methods for identifying gene x environmental interactions
Development of Bayesian methods motivated by applications with complex and high-dimensional data.
A particular focus is on nonparametric Bayes approaches for conditional distributions and for flexible borrowing of information. I am also interested in methods for accommodating model uncertainty in hierarchical models, and in latent variable methods, including structural equation models. A recent interest has been in functional data analysis.
- Areas of Interest:
- Functional data analysis
Genetics Latent variable methods Machine learning Molecular epidemiology Nonparametric Bayes Order restricted inference Model selection and averaging
- Keywords:
- Action Potentials • Algorithms • Artificial Intelligence • B-Lymphocytes • Bayes Theorem • Computer Simulation • Data Interpretation, Statistical • DNA-Binding Proteins • Electrophysiological Phenomena • Epidemiologic Methods • Gene Dosage • Genetic Association Studies • Germinal Center • Longitudinal Studies • Markov Chains • Models, Statistical • Models, Theoretical • Monte Carlo Method • Multivariate Analysis • Neurons • Pattern Recognition, Automated • Phenotype • Probability • Stochastic differential equations
- Representative Publications
(More Publications)
(search)
- Dunson, DB, Nonparametric Bayes local partition models for random effects.,
Biometrika, vol. 96 no. 2
(January, 2009),
pp. 249-262, ISSN 0006-3444 [doi] [abs]
- Bigelow, JL; Dunson, DB, Bayesian semiparametric joint models for functional predictors.,
Journal of the American Statistical Association, vol. 104 no. 485
(January, 2009),
pp. 26-36, Informa UK Limited, ISSN 0162-1459 [doi] [abs]
- Dunson, DB; Xing, C, Nonparametric Bayes Modeling of Multivariate Categorical Data.,
Journal of the American Statistical Association, vol. 104 no. 487
(January, 2012),
pp. 1042-1051, ISSN 0162-1459 [doi] [abs]
- Dunson, DB; Park, JH, Kernel stick-breaking processes,
Biometrika, vol. 95 no. 2
(June, 2008),
pp. 307-323, Oxford University Press (OUP), ISSN 0006-3444 [doi] [abs]
- Dunson, DB; Herring, AH; Engel, SM, Bayesian selection and clustering of polymorphisms in functionally related genes,
Journal of the American Statistical Association, vol. 103 no. 482
(June, 2008),
pp. 534-546, Informa UK Limited, ISSN 0162-1459 [doi] [abs]
- Recent Grant Support
- Improving inferences on health effects of chemical exposures, National Institute of Environmental Health Sciences, 2023/08-2028/05.
- R01: Genetic Origins of Adverse Outcomes in African Americans with Lymphoma, National Institutes of Health, 2023/04-2028/03.
- Clinical and Genetic of Monomorphic Epitheliotropic Intestinal T Cell Lymphoma, National Cancer Institute, 2023/01-2027/12.
- A Planetary Inventory of Life - a New Synthesis Built on Big Data Combined with Novel Statistical Methods, European Research Council, 2020/04-2026/03.
- Science-Integrated Predictive modeLing (SCINPL): a novel framework for scalable and interpretable predictive scientific computing, National Science Foundation, 2022/08-2025/07.
- Duke University Program in Environmental Health, National Institutes of Health, 2019/07-2024/06.
- Calibrated uncertainty quantification in statistical learning, Office of Naval Research, 2021/05-2024/05.
- HDR TRIPODS: Innovations in Data Science: Integrating Stochastic Modeling, Data Representation, and Algorithms, National Science Foundation, 2019/10-2023/09.
- Reproducibility and Robustness of Dimensionality Reduction, National Institutes of Health, 2017/09-2023/07.
- Postdoctoral Training in Genomic Medicine Research, National Institutes of Health, 2017/06-2023/06.
- Structured nonparametric methods for mixtures of exposures, National Institutes of Health, 1R01-ES028804-01, 2018/03-2023/02.
- CRCNS: Geometry-based Brain Connectome Analysis, National Institutes of Health, 1R01-MH118927-01, 2018/09-2022/06.
- An Integrated Nonparametric Bayesian and Deep Neural Network Framework for Biologically-Inspired Lifelong Learning, Defense Advanced Research Projects Agency, 2018/02-2022/03.
|